This surge — fueled by competitive pressure and promises of enhanced customer insights — has institutions like Bank of America allocating $4 billion to AI and other new tech initiatives. While early adopters report efficiency gains and cost reductions, the sector faces a pivotal challenge: The average expected ROI timeline of two years reflects both optimism and pressure to demonstrate quick wins. Success hinges on overcoming fragmented implementations and workforce skepticism that could dilute returns.
The allure of AI-driven efficiency
Within AI budgets, financial institutions are prioritizing data modernization (58% of AI budgets) and licensing generative AI software (53%) to unlock customer insights and streamline operations. These investments aim to address long-standing inefficiencies — from legacy system overhauls to real-time fraud detection. Bank of America’s seven-year AI journey demonstrates this principle. The bank reduced service costs and increased client satisfaction scores by centralizing data from 20 million Erica virtual assistant users.
Yet the focus remains narrow. Nearly two-thirds of institutions view AI primarily as a tool for “bottom-line productivity”, while only 12% have implemented enterprise-wide AI strategies. This myopia risks creating advanced capabilities in silos — a customer service chatbot here, a risk-modeling algorithm there — without cohesive integration. AI governance must be defined as part of enterprise strategy, not an afterthought.
The execution gap: Strategy versus reality
Despite ambitious AI strategies, financial institutions face a stark execution gap. AI progress is threatened by fragmented data, talent shortages, and weak governance.
- Data fragmentation: 58% of AI budgets target data modernization, but 18% of institutions cite poor data quality as a top barrier. Many institutions still wrestle with inconsistent customer data across credit cards, mortgages, and wealth management platforms.
- Talent shortages: There are two pivotal talent issues. One is that talent ranks among the top barriers to AI success — finding, training, and retaining AI talent. Two is the workforce distrust that could derail even technically sound AI initiatives.
- Governance vacuum: Only 23% of institutions have mature AI governance frameworks, leaving many unable to address model bias or explainability concerns.
These challenges compound when viewed through an organizational lens. With 34% of AI strategies defined at regional levels, a European bank’s chatbot project, for example, might use data protocols different from those of its American counterpart’s credit scoring model, limiting scalability.
The human factor: trust as a make-or-break variable
One of the great fallacies of the AI talent conundrum is that AI execution only requires technical or data science experience. However, the solution extends beyond hiring data scientists. The required talent mix covers strategy, technology, engineering, data science, business process, and risk and compliance. While AI technical talent is critical to cultivate, financial institutions should take their employees on the AI journey by upskilling them to use and benefit from AI investments. In the future, all talent must be AI talent. AI literacy will be essential — not just for specialists, but across all roles to effectively collaborate with, manage, and make the best use of AI-driven tools and insights.
Frontline employees resistant to algorithm-driven loan approvals or relationship managers skeptical of AI-generated client advice create adoption friction. AI’s potential falters without employee buy-in. Institutions reporting high AI adoption must:
- Demystify AI: Financial institutions can assist their employees through transparent model documentation and employee co-creation workshops
- Transparent upskilling: Bank of America’s Academy, the bank’s training arm, has turned to artificial intelligence to sharpen staff skills. Through AI-powered conversation simulators, employees rehearse client interactions and receive instant feedback. Last year, staff completed over a million such simulations, with many reporting that this practice leads to more consistent and higher-quality service.
- Measure trust metrics: These metrics gauge how comfortable staff rely on AI outputs for decision-making, such as credit underwriting or customer advice. One research found that organizations with higher AI trust conduct regular reviews of AI outputs — 74% of successful companies check AI results at least weekly — ensuring oversight and improving confidence.
- Ethical governance frameworks: Institutions with clear AI bias mitigation protocols report 28% higher workforce trust scores.
Strategic imperatives for AI-first leadership
To avoid becoming cautionary tales, financial institutions must:
- Align AI spending with business outcomes: Tie data modernization projects to specific revenue goals. They must also phase generative AI deployments from low-risk areas (marketing content generation) to core processes (regulatory reporting).
- Institutionalize AI governance: Banks can establish cross-functional councils to oversee model ethics and compliance. Implementing real-time monitoring for AI-driven decisions such as loan denials can also help with governance.
- Bridge the talent gap: Focusing on AI literacy, creating “AI translator” roles to mediate between technical teams and business units, and providing explainable decisions by high-impact AI systems.
- Prioritize use case alignment: McKinsey found that tracking institutions linking AI projects to specific KPIs generated the most impact on their bottom lines.
Unlocking AI’s potential requires dismantling silos between IT spending and business value. Institutions that marry technological ambition with organizational trust-building will likely move ahead. In this high-stakes transition, the ultimate metric won’t be algorithms deployed or dollars spent but sustained alignment between silicon and human intelligence. The race isn’t for the biggest budget, but for the most coherent strategy.
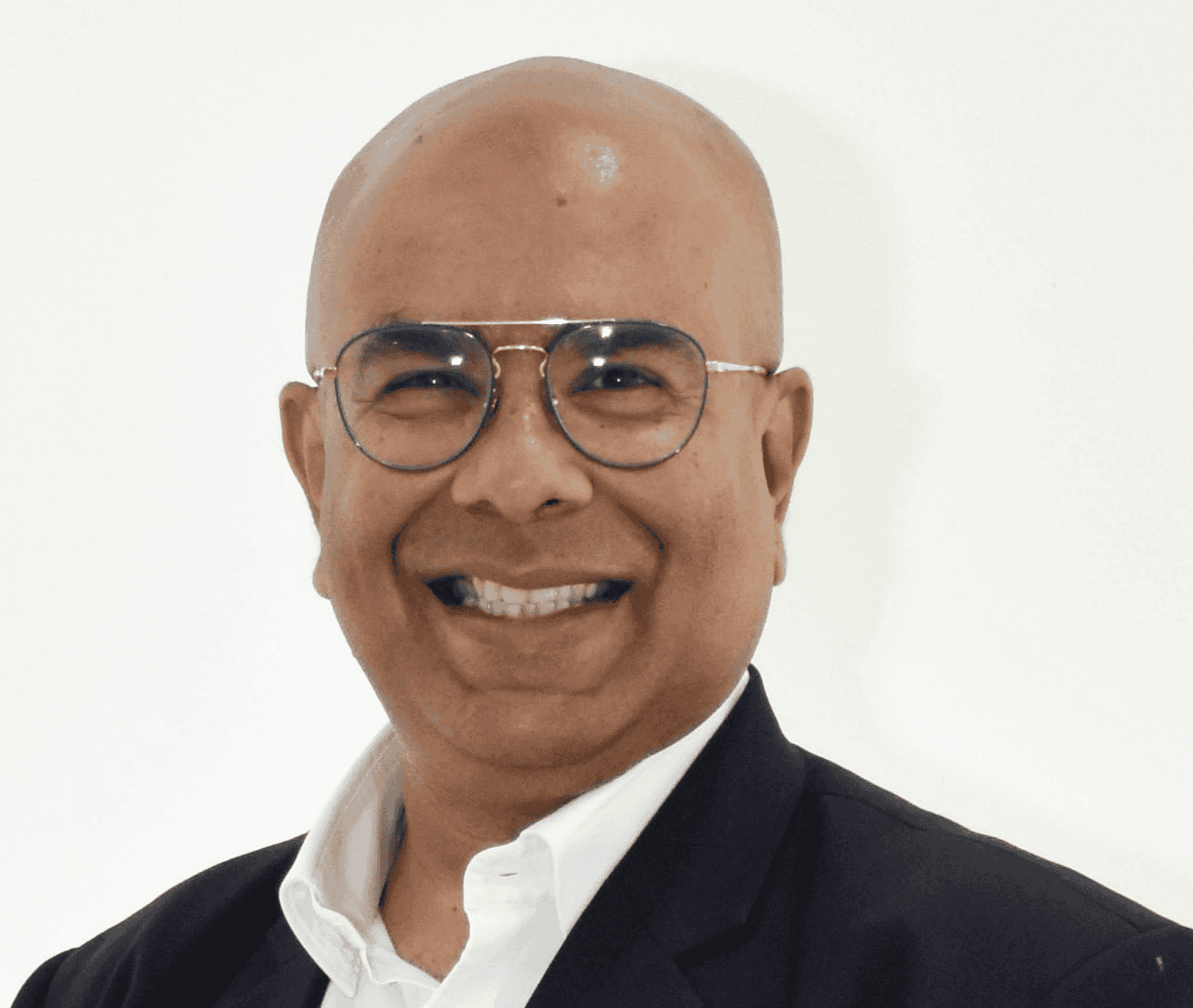
Jay Nair
Executive Vice President and Industry Head for Financial Services in Europe, Middle East, and Africa| Infosys
About The Author
Jay Nair is the Executive Vice President and Industry Head for Financial Services in Europe, Middle East, and Africa. Additionally, he leads the UK Public Service business for Infosys. He is also part of the Supervisory board for Stater.ni (which is largest independent end-to-end service provider for the mortgage market in the Benelux).
He has spent close to three decades in Engineering -both in process control engineering and since 1999, within the BFSI (Banking, Financial Services and Insurance) sector. Jay has extensive experience in Business and Technology Consulting, Practice development, Engineering and Largescale enterprise-wide technology program management. He has led global teams and programs around in the Americas ,Europe ,India, China ,LATAM, and the Asia Pacific.
He has post graduate qualifications in both Software Engineering as well as Business Management.